Deep Generative Models
- DR.GEEK
- Nov 28, 2020
- 1 min read
(28th-Nov-2020)
• Present several of the specific kinds of generative models that can be built and trained using the techniques presented in chapters – . All of 16 19 these models represent probability distributions over multiple variables in some way. Some allow the probability distribution function to be evaluated explicitly. Others do not allow the evaluation of the probability distribution function, but support operations that implicitly require knowledge of it, such as drawing samples from the distribution. Some of these models are structured probabilistic models described in terms of graphs and factors, using the language of graphical models presented in chapter . Others can not easily be described in terms of factors, 16 but represent probability distributions nonetheless.
• Boltzmann Machines
• Boltzmann machines were originally introduced as a general “connectionist” approach to learning arbitrary probability distributions over binary vectors (Fahlman et al., ; 1983 Ackley 1985 Hinton 1984 Hinton and Sejnowski 1986 et al., ; et al., ; , ). Variants of the Boltzmann machine that include other kinds of variables have long ago surpassed the popularity of the original. In this section we briefly introduce the binary Boltzmann machine and discuss the issues that come up when trying to train and perform inference in the model. We define the Boltzmann machine over a d-dimensional binary random vector x ∈{0,1}d. The Boltzmann machine is an energy-based model (section ), 16.2.4 meaning we define the joint probability distribution using an energy function:
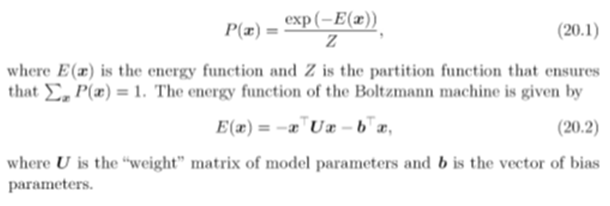
Comments