Generative Adversarial Networks
- DR.GEEK
- Dec 15, 2020
- 1 min read
(15th-December-2020)
• Generative adversarial networks or GANs ( , ) are another Good fellow et al. 2014c generative modeling approach based on differentiable generator networks. Generative adversarial networks are based on a game theoretic scenario in which the generator network must compete against an adversary. The generator network directly produces samplesx = g(z;θ( ) g ). Its adversary, the discriminator network, attempts to distinguish between samples drawn from the training data and samples drawn from the generator. The discriminator emits a probability value given by d(x;θ( ) d ), indicating the probability that x is a real training example rather than a fake sample drawn from the model. The simplest way to formulate learning in generative adversarial networks is as a zero-sum game, in which a function v(θ( ) g ,θ( ) d ) determines the payoff of the discriminator. The generator receives −v(θ( ) g ,θ( ) d ) as its own payoff. During learning, each player attempts to maximize its own payoff, so that at convergence


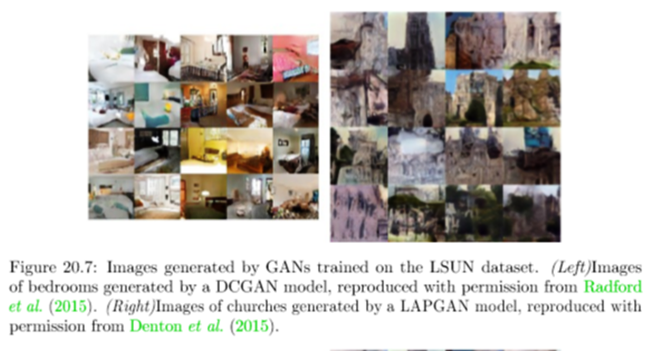
Comments