Interesting Properties
- DR.GEEK
- Dec 3, 2020
- 2 min read
(3rd-Dec-2020)
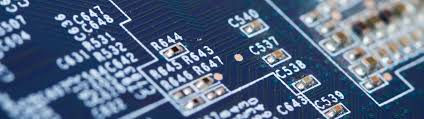
• Deep Boltzmann machines have many interesting properties. DBMs were developed after DBNs. Compared to DBNs, the posterior distribution P(h v | ) is simpler for DBMs. Somewhat counterintuitively, the simplicity of this posterior distribution allows richer approximations of the posterior. In the case of the DBN, we perform classification using a heuristically motivated approximate inference procedure, in which we guess that a reasonable value for the mean field expectation of the hidden units can be provided by an upward pass through the network in an MLP that uses sigmoid activation functions and the same weights as the original DBN. distribution Any Q(h) may be used to obtain a variational lower bound on the log-likelihood. This heuristic procedure therefore allows us to obtain such a bound. However, the bound is not explicitly optimized in any way, so the bound may be far from tight. In particular, the heuristic estimate of Q ignores interactions between hidden units within the same layer as well as the top-down feedback influence of hidden units in deeper layers on hidden units that are closer to the input. Because the heuristic MLP-based inference procedure in the DBN is not able to account for these interactions, the resulting Q is presumably far from optimal. In DBMs, all of the hidden units within a layer are conditionally independent given the other layers.
• This lack of intralayer interaction makes it possible to use fixed point equations to actually optimize the variational lower bound and find the true optimal mean field expectations (to within some numerical tolerance). The use of proper mean field allows the approximate inference procedure for DBMs to capture the influence of top-down feedback interactions. This makes DBMs interesting from the point of view of neuroscience, because the human brain is known to use many top-down feedback connections. Because of this property, DBMs have been used as computational models of real neuroscientific phenomena ( , ; Series et al. 2010 Reichert 2011 et al., ). One unfortunate property of DBMs is that sampling from them is relatively difficult. DBNs only need to use MCMC sampling in their top pair of layers. The other layers are used only at the end of the sampling process, in one efficient ancestral sampling pass. To generate a sample from a DBM, it is necessary to use MCMC across all layers, with every layer of the model participating in every Markov chain transition.
Comentários