(24th-June-2020)
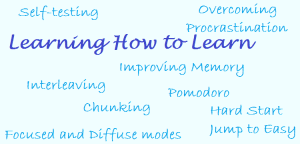
• Learning is the ability of an agent improve its behavior based on experience.
• Supervised learning is the problem that involves predicting the output of a new input, given a set of input-output pairs.
• Given some training examples, an agent builds a representation that can be used for new predictions.
• Linear classifiers, decision trees, and Bayesian classifiers are all simple representations that are the basis for more sophisticated models.
• An agent can choose the best hypothesis given the training examples, delineate all of the hypotheses that are consistent with the data, or compute the posterior probability of the hypotheses given the training examples.
Approaches and algorithms
Analytical learning
Artificial neural network
Backpropagation
Boosting (meta-algorithm)
Bayesian statistics
Case-based reasoning
Decision tree learning
Inductive logic programming
Gaussian process regression
Group method of data handling
Kernel estimators
Learning Automata
Learning Classifier Systems
Minimum message length (decision trees, decision graphs, etc.)
Multilinear subspace learning
Naive bayes classifier
Maximum entropy classifier
Conditional random field
Nearest Neighbor Algorithm
Probably approximately correct learning (PAC) learning
Ripple down rules, a knowledge acquisition methodology
Symbolic machine learning algorithms
Subsymbolic machine learning algorithms
Support vector machines
Minimum Complexity Machines (MCM)
Random Forests
Ensembles of Classifiers
Ordinal classification
Data Pre-processing
Handling imbalanced datasets
Statistical relational learning
Proaftn, a multicriteria classification algorithm
Comments