Probably Approximately Correct Learning
- DR.GEEK
- Jun 14, 2020
- 2 min read
(20th-June-2020)
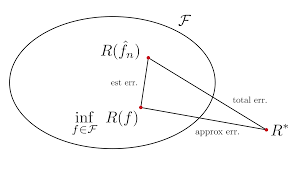
• So far, we have seen a number of different learning algorithms. This section covers some of the theoretical aspects of learning, developed in an area called computational learning theory.
• Some relevant questions that we can ask about a theory of computational learning include the following:
• Is the learner guaranteed to converge to the correct hypothesis as the number of examples increases?
• How many examples are required to identify a concept?
• How much computation is required to identify a concept?
• In general, the answer to the first question is "no," unless it can be guaranteed that the examples always eventually rule out all but the correct hypothesis. Someone out to trick the learner could choose examples that do not help discriminate correct hypotheses from incorrect hypotheses. So if such a person cannot be ruled out, a learner cannot guarantee to find a consistent hypothesis. However, given randomly chosen examples, a learner that always chooses a consistent hypothesis can get arbitrarily close to the correct concept.
• For example, Suppose the hypothesis space H is the set of conjunctions of literals on n Boolean variables. In this case |H|=3n+1 because, for each conjunction, each variable in is one of three states: (1) it is unnegated in the conjunction, (2) it is negated, or (3) it does not appear; the "+1" is needed to represent false, which is the conjunction of any atom and its negation. Thus, the sample complexity is (1)/(ε)(nln3 + ln(1)/(δ)) examples, which is polynomial in n, (1)/(ε), and ln(1)/(δ).
• If we want to guarantee at most a 5% error 99% of the time and have 30 Boolean variables, then ε= 1/20, δ=1/100, and n=30. The bound says that we can guarantee this performance if we find a hypothesis that is consistent with 20×(30ln3 + ln100) approx 752 examples. This is much less than the number of possible instances, which is 230=1,073,741,824, and the number of hypotheses, which is 330+1=205,891,132,094,650.
Comments