Variable Elimination for Belief Networks
- DR.GEEK
- Sep 5, 2020
- 2 min read
(5th-September-2020)
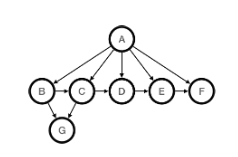
• This section gives an algorithm for finding the posterior distribution for a variable in an arbitrarily structured belief network. Many of the efficient exact methods can be seen as optimizations of this algorithm. This algorithm can be seen as a variant of variable elimination (VE) for constraint satisfaction problems (CSPs) or VE for soft constraints.
• The algorithm is based on the notion that a belief network specifies a factorization of the joint probability distribution. Before we give the algorithm, we define factors and the operations that will be performed on them. Recall that P(X|Y) is a function from variables (or sets of variables) X and Y into the real numbers that, given a value for X and a value for Y, gives the conditional probability of the value for X, given the value for Y. This idea of a function of variables is generalized as the notion of a factor. The VE algorithm for belief networks manipulates factors to compute posterior probabilities.
Many problems are too big for exact inference, and one must resort to approximate inference. One of the most effective methods is based on generating random samples from the (posterior) distribution that the network specifies.
Stochastic simulation is based on the idea that a set of samples can be used to compute probabilities. For example, you could interpret the probability P(a)=0.14 as meaning that, out of 1,000 samples, about 140 will have a true. You can go from (enough) samples into probabilities and from probabilities into samples.
We consider three problems:
how to generate samples,
how to incorporate observations, and
how to infer probabilities from samples.
We examine three methods that use sampling to compute the posterior distribution of a variable: (1) rejection sampling, (2) importance sampling, and (3) particle filtering.
Comments