What can you do with an NN and what not?
- DR.GEEK
- Jan 26, 2021
- 1 min read
(26th-January-2021)
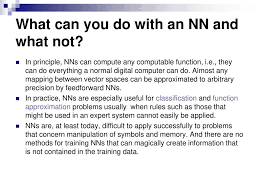
• In principle, NNs can compute any computable function, i.e., they can do everything a normal digital computer can do. Almost any mapping between vector spaces can be approximated to arbitrary precision by feedforward NNs
• In practice, NNs are especially useful for classification and function approximation problems usually when rules such as those that might be used in an expert system cannot easily be applied.
• NNs are, at least today, difficult to apply successfully to problems that concern manipulation of symbols and memory. And there are no methods for training NNs that can magically create information that is not contained in the training data.
• Who is concerned with NNs?
• Computer scientists want to find out about the properties of non-symbolic information processing with neural nets and about learning systems in general.
• Statisticians use neural nets as flexible, nonlinear regression and classification models.
• Engineers of many kinds exploit the capabilities of neural networks in many areas, such as signal processing and automatic control.
• Cognitive scientists view neural networks as a possible apparatus to describe models of thinking and consciousness (High-level brain function).
• Neuro-physiologists use neural networks to describe and explore medium-level brain function (e.g. memory, sensory system, motorics).
• Physicists use neural networks to model phenomena in statistical mechanics and for a lot of other tasks.
• Biologists use Neural Networks to interpret nucleotide sequences.
• Philosophers and some other people may also be interested in Neural Networks for various reasons
Comments